Investigating the Impact of Media Bias on News Readers
By Delphine Gardiner, Amir Tohidi, Samar Haider
In recent years, especially following the 2016 presidential election, both the media and American society have been grappling with the pervasive issue of fake news. This has sparked a surge in related research (Lazer et al., 2018; Vosoughi, Roy, Aral, 2018). However, spreading false information is not the only way to influence public opinion. For example, Allen et al. (2024) demonstrated that factually accurate but misleading content on Facebook can significantly cast doubts on vaccine safety or efficacy, 46 times more than posts flagged as misinformation by third-party fact-checkers.
Mainstream media can also mislead the public through their selective choice of facts, or framing of their narratives, even without disseminating outright falsehoods. While these tactics are extensively studied in Political Science and Communications, advancements in Large Language Models (LLMs) offer new tools to test their impact in a controlled environment. Motivated by journalistic choices and their influence on readers, Amir Tohidi and Samar Haider used data from the Media Bias Detector dashboard to extract information from actual news articles on various events. They then used LLMs to generate synthetic articles with carefully controlled biases and assessed the impact of these articles on people through a survey experiment.
They presented their work, “Investigating the Impact of Media Bias on News Readers,” at the 2024 International Conference on Computational Social Sciences (IC2S2) which was held at the University of Pennsylvania last week.
In particular, Tohidi and Haider study the impact of selection and framing. In this context, selection refers to the choice of which facts to include or omit in an article while framing refers to how they are presented, i.e. how they are ordered and what kind of tone or emphasis is placed on them. Framing can also include the use of opinions and quotes to reinforce a particular view. This selection and framing by journalists on both sides of the political spectrum creates different “realities” for their readers as they end up with very different perspectives of the same topic depending on the news sources they rely on.
They then developed a pipeline to create GPT-generated articles which would reflect the different uses of selection and framing in the media. Tohidi and Haider used the bias detector events data to identify substantial events over time and collect all relevant articles written about them by different publishers. Their pilot study focused on the Federal Reserve’s announcement of increased interest rates in March 2023. As part of the bias detector pipeline, all articles related to this event were run through GPT-4 which was instructed to label every sentence in them by type (fact, opinion, or quote) and tone (positive, negative, or neutral). You can find further details in the dashboard’s methodology page.
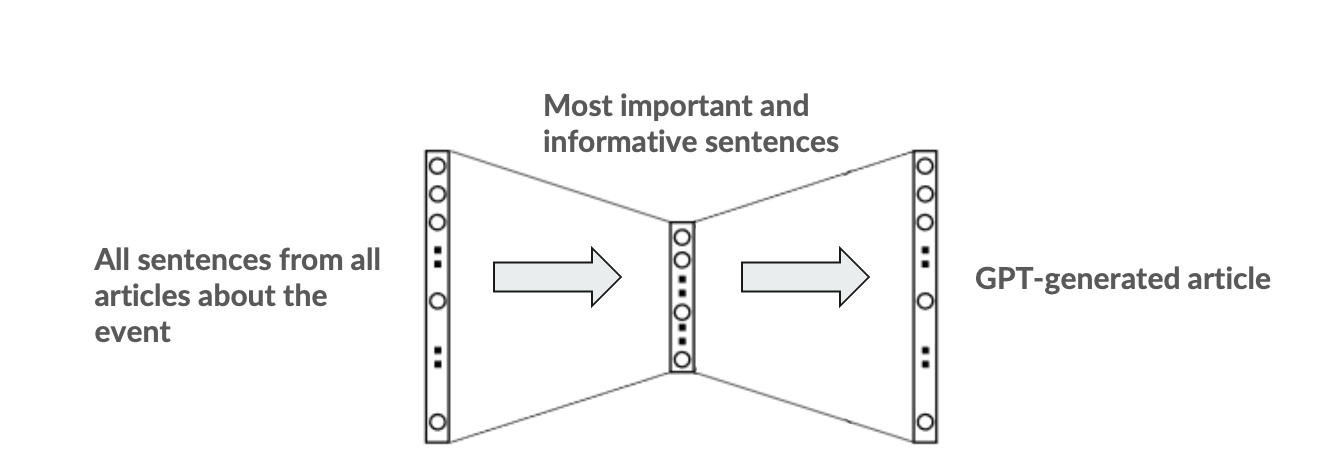
Once a large enough set of facts about an event had been collected from different articles, they were clustered together based on their semantic similarity to remove identical statements and keep only the most representative and informative ones from each bucket. This was to minimize repeated statements while also maximizing the diversity of information being used as input for the article generation step.
With these final groups of sentences, Tohidi and Haider asked GPT to generate multiple articles by varying the selection and framing dimensions. To vary the selection, they changed the set of input factual statements that GPT used to write the article. For instance, for positive selection, GPT was given 16 positive facts, whereas for negative selection, it was given 16 negative facts. To alter the framing, they combined quotes, opinions, and GPT’s ability to write in a particular tone. For example, for positive framing, the model received four positive quotes and opinions and was instructed to write the story with an overall positive tone. The specific prompts used for this task are illustrated in Figure 2. These variations result in a 3x3 experimental design which is illustrated in Figure 3 (The Negative Selection & Positive Framing, and Positive Selection & Negative Framing conditions are unrealistic in practice, so were removed from the experimental conditions).
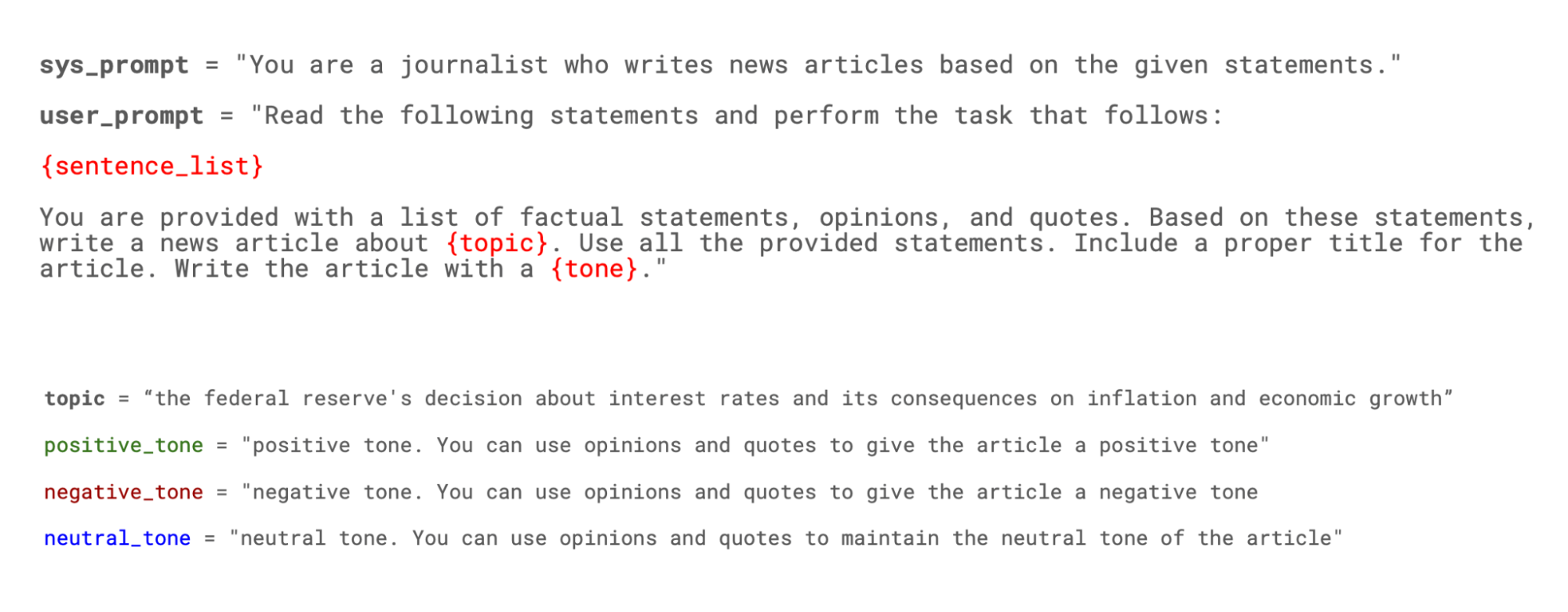
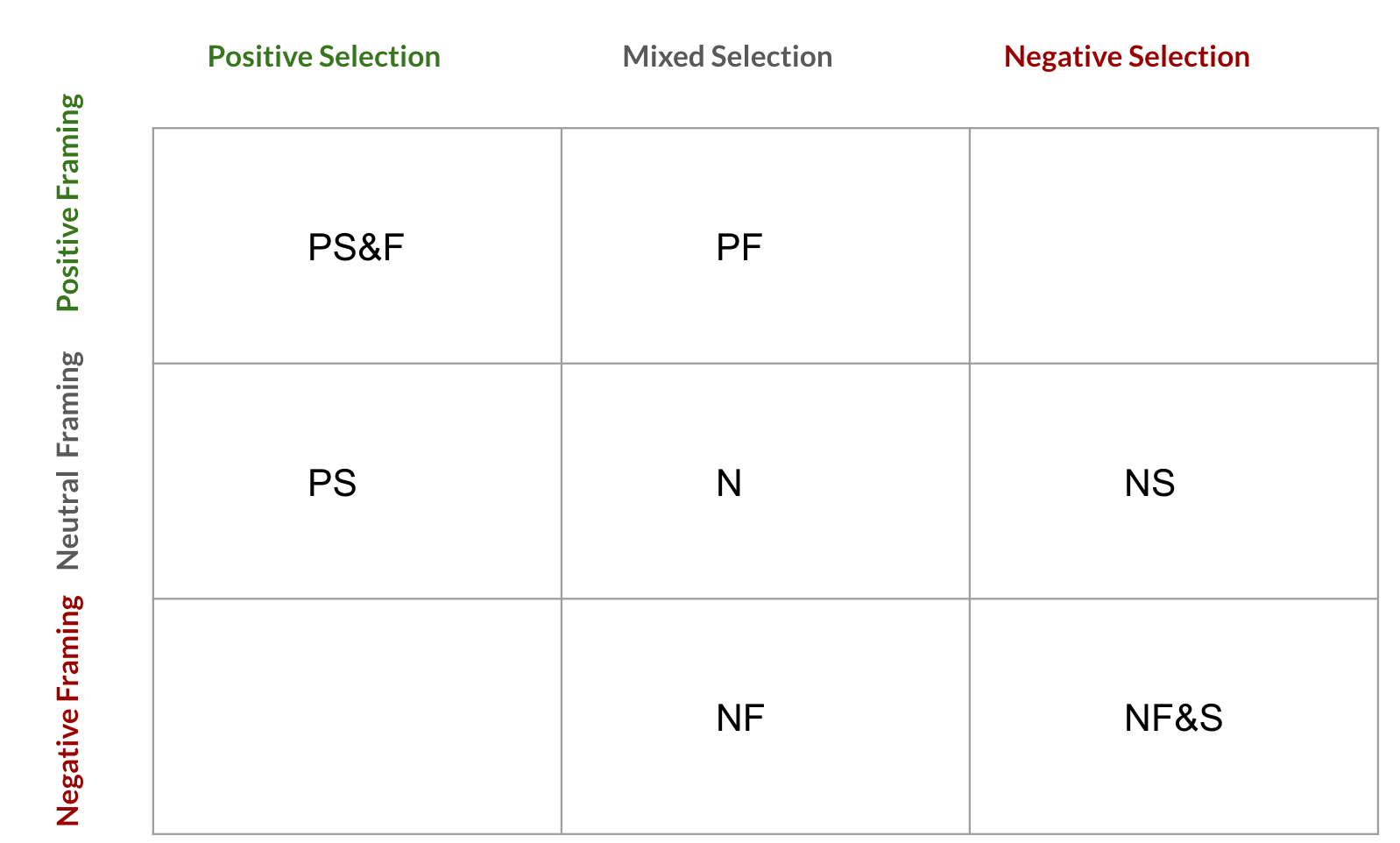
Finally, these articles were tested in an online randomized survey experiment, where human participants were asked to read one of these variations of the news article and then provide answers to a set of related questions. Tohidi and Haider found that even a one-time exposure to news articles containing a slight selection or framing bias could significantly influence people’s perceptions of the same topic. Notably, news that skewed negative had a stronger impact on public opinion compared to positive news.
The ability of LLMs to generate realistic text opens up a new paradigm of studies that were not possible before. By using them to write artificial news articles which are constrained to the same set of facts but framed in different ways, we can study the impact of this framing bias in a controlled setting. This approach allows researchers to gain insight into the subtle ways in which media bias can influence readers, while also helping journalists understand the sometimes unintended effects of the decisions they make when writing news articles. Tohidi’s and Haider’s findings from this work highlight the importance of subtle but prevalent biases in media, which are captured and revealed in the Media Bias Detector.